By Sara Bauder
Unbiased research is really at the heart of all the extension information we provide the public. Often times researchers use statistical terminology and big data sets to make conclusions, this can often times make data difficult for the reader to interpret.
In order to be considered “sound” research, replication and randomization is vital to a project. When setting up a field study, it might be tempting to split a field in half, but this approach can end with misleading results because variability exists across a field due to many factors. Variability can be caused by differences in soil type, topography, management practices, drainage, pesticide residues, plant disease, soil compaction, weather, and more. To mitigate these issues in the field setting and create a somewhat equal playing field for all treatments being tested, randomization and replication are used.
Replication and Randomization
Replication simply means that each treatment (factor being tested) is repeated more than one time across a study area, typically 3-6 times in agriculture field research. Within each replication, randomization is also practiced; this means that treatments are randomly placed within each replication. This assures that treatment performance is not based upon location in the field. Although every research trial has some degree of error, replication and randomization protect research trials from a number of factors that could skew data for reasons other than those being tested.
Replication and randomization also allow for statistics to be completed on experiments. When someone says a research result is ‘significant’, it actually means that it has been deemed statistically different from another treatment result. As business operators, we want to use practices that have a high likelihood of paying off; statistics and sound research help us determine the probability of a particular practice or treatment that influences yield (or any factor/value tested) versus other outside factors of error and variability.
Least Significant Difference
A term commonly used in research is the ‘least significant difference’ or ‘LSD’; it is used to compare means of different treatments. For example, in a hybrid variety trial, the LSD is the minimum bushels per acre that two hybrids must differ by before we could consider them to be ‘significantly different’. Note there is no way to calculate LSD if a field is simply split in half; randomization and replication are needed. In ag research, LSD is often calculated at the 0.05 or 0.10 significance level. For example, the 0.05 significance level means you can be 95% certain that the treatments being tested really did differ in yield if the difference between them was equal to or greater than the LSD.
Often times, people wonder how two numbers that look different can be considered ‘not significantly different’. Results from an example trial demonstrate the importance of statistical analysis in helping determine if yield differences are ‘real’. For example, three crop management systems were evaluated at several locations over three years. Treatments were randomized and replicated four times at each location (Table 1). At the site, the average corn yield for treatment 1 was 10bu/ac greater than in treatment 3. Yield was not statistically significant, however, so we cannot conclude that one management system is higher yielding than the other.
TABLE 1. EXAMPLE FIELD TRIAL.
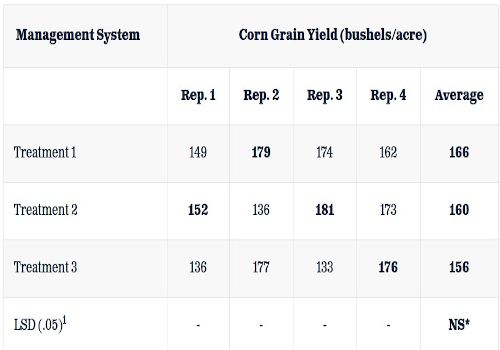
1 If the LSD is not significant (NS) in a trial it is not typically listed- such as in this case;
if it was 10 or less, it would have been listed, as data would have had significant differences.
When looking closer, this data shows that while treatment 1 out-yielded treatment 3 in the first replication, treatment 3 out-yielded treatment 1 by 14 bu/ac in the fourth replication. Due to variabilities like these, statistical analysis showed that we could not say with confidence that any management system was consistently higher yielding than another at this site, thus showing the importance of statistical analysis.
The Bottom Line
When treatments are not described as statistically significant, we should not treat them that way. There are many factors that can cause a treatment to under or over perform; this is why statistically sound research is so important to making good management decisions. Although research results and statistical terminology can seem overwhelming, understanding the basic concepts can be quite valuable for decision making. There is a multitude of information and products available on the market. When making management decisions or purchases, don’t hesitate to ask for research results and statistics to back up marketing claims.
Source : sdstate.edu