Although beef is one of the most consumed foods around the world, eating it when it's past its prime is not only unsavory, but also poses some serious health risks. Unfortunately, available methods to check for beef freshness have various disadvantages that keep them from being useful to the public. For example, chemical analysis or microbial population evaluations take too much time and require the skills of a professional. On the other hand, non-destructive approaches based on near-infrared spectroscopy require expensive and sophisticated equipment. Could artificial intelligence be the key to a more cost-effective way to assess the freshness of beef?
At Gwangju Institute of Science and Technology (GIST), Korea, a team of scientists led by Associate Processors Kyoobin Lee and Jae Gwan Kim have developed a new strategy that combines deep learning with diffuse reflectance spectroscopy (DRS), a relatively inexpensive optical technique. "Unlike other types of spectroscopy, DRS does not require complex calibration; instead, it can be used to quantify part of the molecular composition of a sample using just an affordable and easily configurable spectrometer," explains Lee. The findings of their study are now published in Food Chemistry.
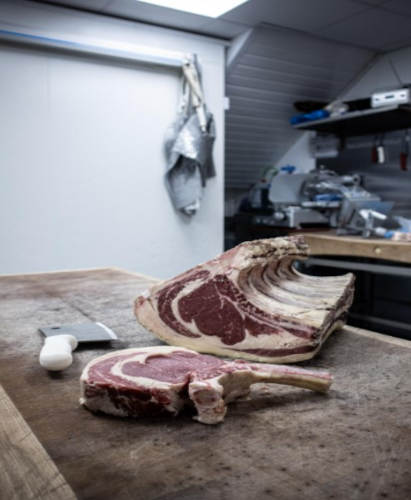
Consuming spoiled beef is dangerous, but there are currently no simple and efficient methods to assess beef freshness.
To determine the freshness of beef samples, they relied on DRS measurements to estimate the proportions of different forms of myoglobin in the meat. Myoglobin and its derivatives are the proteins mainly responsible for the color of meat and its changes during the decomposition process. However, manually converting DRS measurements into myoglobin concentrations to finally decide upon the freshness of a sample is not a very accurate strategy--and this is where deep learning comes into play.
Convolutional neural networks (CNN) are widely used artificial intelligence algorithms that can learn from a pre-classified dataset, referred to as 'training set,' and find hidden patterns in the data to classify new inputs. To train the CNN, the researchers gathered data on 78 beef samples during their spoilage process by regularly measuring their pH (acidity) alongside their DRS profiles. After manually classifying the DRS data based on the pH values as 'fresh,' 'normal,' or 'spoiled,' they fed the algorithm the labelled DRS dataset and also fused this information with myoglobin estimations. "By providing both myoglobin and spectral information, our trained deep learning algorithm could correctly classify the freshness of beef samples in a matter of seconds in about 92% of cases," highlights Kim.
Click here to see more...